Iceberg, and the elephant in the room
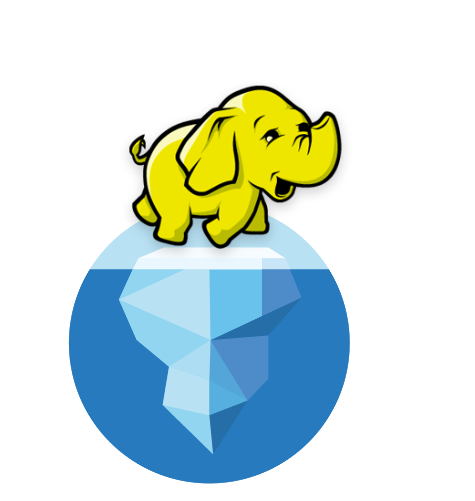
Happy new year everyone! If you had a break over the holidays I hope you had a good one.
The start of the year is often a time to refresh (or create) data strategies and technical roadmaps.
How many technical roadmaps will include adoption of Apache Iceberg?
This recent post on how Iceberg adoption is reminiscent of Hadoop adoption really resonated with me, with people rushing to adopt it without having the right organisational maturity.
All this reminds me of a data platform I worked on about 10 years ago.
Our architect directed us to adopt Hadoop and move the source of data from a Vertica database to HDFS.
But to do so we needed a load of other tooling. Mesos for cluster management. Chronos for scheduling. Hadoop/Pig (and a bit later, Spark) for querying. And so on.
We were only a small team, and now instead of delivering value we were spending our time looking after all this infrastructure! Infrastructure that was designed to solve the problems of big tech—problems we didn’t have (Hadoop came from Yahoo; Mesos & Chronos was used heavily at Twitter).
We also adopted a new architecture to make use of this new tech, the lambda architecture, and rewrote a load of services to move it to this architecture.
All of this effort, and we didn’t need to do any of it!
It didn’t solve any of the problems we had, and our team drowned in the added complexity of the new stack and architecture.
I learned a lot from that experience. I’m now very sceptical of brining in new tech, new architectures, etc, until I’m certain it solves our problems.
Iceberg is cool tech. I can imagine it solved a number of problems at Netflix, where it originated.
But I’m not yet certain Iceberg solves the problems most of us have.
Just like Hadoop didn’t.
Interesting links
Data Contracts, the game by Joe Leach
I love this! A really innovative way to explain data contracts and get people engaging with how to use them to correctly categorise and set expectations of data.
I have found that data owners can struggle to understand why this is important, and then how to decide how data should be categorised for safe data management and monitored against SLOs. Something like this could really help with that.
Databases in 2024: A Year in Review by Andy Pavlo
Interesting read on databases, vendor competition, and licensing.
Though see above before adopting a new database :)
DuckDB is another one where for a long time I felt it was a solution looking for problems to solve, but I’m starting to agree that many organisations don’t need a big cloud-based data warehouse like BigQuery, Snowflake, etc, and can save a lot of money by using DuckDB instead.
Even larger organisations might benefit from a “multi-engine data stack”, where some workloads are offloaded from the data warehouse to DuckDB. However, adding another engine and changing your architecture to take advantage of it does add a lot of complexity, so the savings need to be worth it.
Being punny 😅
WANTED: A man has been stealing wheels off of police cars. Police are working tirelessly to catch him.
Upcoming workshops
- Implementing a Data Mesh with Data Contracts - Antwerp, Belgium - June 5 2025
- Alongside the inaugural Data Mesh Live conference
- Sign up here
Thanks! If you’d like to support my work…
Thanks for reading this weeks newsletter - always appreciated!
If you’d like to support my work consider buying my book, Driving Data Quality with Data Contracts, or if you have it already please leave a review on Amazon.
Enjoy your weekend.
Andrew