The democratisation of Data Science
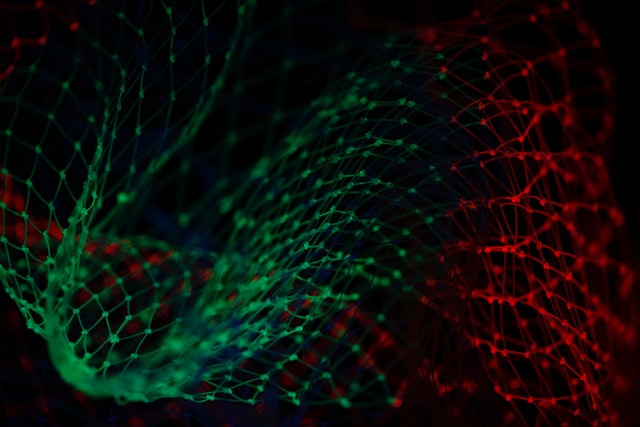
There’s a trend in the industry to make data science and machine learning more accessible, allowing engineers to build and deploy standard models without needing to have a strong data science background. Examples include BigQueryML (standard models in SQL), Ubers Ludwig and h2o.ai.
BI Analysts and Data Engineers are the most ready to take advantage of these tools, as they have the expertise around data modelling and the domain knowledge of their organisations data. But there’s no reason why any engineer couldn’t make use of these tools for their data.
What’s likely missing at the moment is a primer on when to use machine learning and what kind of problems can it solve for you. Many tutorials focus on areas like image recognition, which are unlikely to be a problem you’ll face in your organisation. What’s more interesting is how machine learning can help you manage your infrastructure, improve customer conversion, and drive features in your applications.
Whilst these kinds of tools will never likely be able to power the most complex and fine-tuned use cases, they do allow teams to experiment with machine learning models, and if the results are good deploy a solution into production. In some of these areas a data scientist may take over and build a more optimised custom model, but in many areas you will have more than good enough results with very little effort.
I’m looking forward to watching these tools evolve over the next few years, and finding areas where they can have great impact with significantly less effort and cost than a dedicated model.
Cover image from Unsplash.